優雅堂本人確認
<ウェブサイト名>
<現在の時刻>
About Overview Organization & Management Strategy Open Positions Movie Library Access Research Structure Researchers & Laboratories Research Areas Three Advanced Target Projects Collaboration Achievements Press Releases Media & Award AIMResearch Publications Topics News Seminars & Symposium International Satellites International Partner Institutions Inter-Faculty Exchange Agreements Researcher Exchange Programs Support Support Systems For International Researchers (IAC) For Visitors Researchers for Visitors for Researchers for Enterprise Access AIMR Fund Japanese Press Releases Machine Learning Guides Carbon Nanotechnology 02/01/2024 Carbon nanostructures could become easier to design and synthesize thanks to a machine learning method that predicts how they grow on metal surfaces. The new approach, developed by researchers at Japan’s Tohoku University and China’s Shanghai Jiao Tong University, will make it easier to exploit the unique chemical versatility of carbon nanotechnology. The method was published in the journal Nature Communications. The growth of carbon nanostructures on a variety of surfaces, including as atomically thin films, has been widely studied, but little is known about the dynamics and atomic-level factors governing the quality of the resulting materials. “Our work addresses a crucial challenge for realizing the potential of carbon nanostructures in electronics or energy processing devices,” says Hao Li of the Tohoku team. Schematic illustrations of carbon-growth-on-metal machine-learning potential (CGM-MLP) generated by active learning on-the-fly during hybrid molecular dynamics and time-stamped force-biased Monte Carlo (MD/tfMC) simulations. ©Hao Li et al. The wide range of possible surfaces and the sensitivity of the process to several variables make direct experimental investigation challenging. The researchers therefore turned to machine learning simulations as a more effective way to explore these systems. With machine learning, various theoretical models can be combined with data from chemistry experiments to predict the dynamics of carbon crystalline growth and determine how it can be controlled to achieve specific results. The simulation program explores strategies and identifies which ones work and which don’t, without the need for humans to guide every step of the process. The researchers tested this approach by investigating simulations of the growth of graphene, a form of carbon, on a copper surface. After establishing the basic framework, they showed how their approach could also be applied to other metallic surfaces, such as titanium, chromium and copper contaminated with oxygen. CGM-MLP driven simulations of graphene growth on Cu(111) with different carbon incident kinetic energies (Ek). (a) 2.5 eV, (b) 5.0 eV, (c) 7.5 eV, and (d) 10 eV. ©Hao Li et al. The distribution of electrons around the nuclei of atoms in different forms of graphene crystals can vary. These subtle differences in atomic structure and electron arrangement affect the overall chemical and electrochemical properties of the material. The machine learning approach can test how these differences affect the diffusion of individual atoms and bonded atoms and the formation of carbon chains, arches and ring structures. The team validated the results of the simulations through experiments and found that they closely matched. “Overall, our work provides a practical and efficient method for designing metallic or alloy substrates to achieve desired carbon nanostructures and explore further opportunities,” Li says. He adds that future work will build on this to investigate topics such as the interfaces between solids and liquids in advanced catalysts and the chemical properties of materials used for processing and storing energy. Representative metallic surfaces for the growth of carbon nanostructures. (a) pure Cu(111), (b) Cr(110, and (c) Ti(001) surface. Below each surface, High resolution transmission electron microscopy (HRTEM) images and selected area electron diffraction (SAED) images of carbon nanostructures prepared by magnetron sputtering deposition are provided. (d) The number of sp2-C as a function of deposited carbon atoms on different metal substrates and e O-contaminated Cu(111). ©Hao Li et al. Publication Details Title: Active Machine Learning Model for the Dynamic Simulation and Growth Mechanisms of Carbon on Metal Surface Authors: Di Zhang*, Peiyun Yi, Xinmin Lai, Linfa Peng*, Hao Li* Journal: Nature Communications DOI: 10.1038/s41467-023-44525-z Contact Hao LiAdvanced Institute for Materials Research (WPI-AIMR), Tohoku University E-mail: li.hao.b8&#64;tohoku.ac.jp Webstie: Hao Li Laboratory Tweet Achievements Press Releases 2024 2023 2022 2021 2020 2019 2018 2017 2016 2015 2014 2013 2012 2011 2010 2009 Media & Award 2024 2023 2022 2021 2020 2019 2018 2017 2016 2015 2014 2013 2012 2011 2010 2009 AIMResearch About AIMResearch Research Highlights 2024 2023 2022 2021 2020 2019 2018 2017 2016 2015 2014 2013 2012 2011 2010 2009 In the Spotlight 2019 2018 2017 2016 2015 2014 2013 2012 2011 2010 2009 Email Alert Sign up Publications Headlines 05/22/2024 Machine Learning Accelerates Discovery o... 05/16/2024 New Data-Driven Model Rapidly Predicts D... 05/15/2024 Researchers Unlock Vital Insights into M... Home Achievements Press Releases 2024 Machine Learning Guides Carbon Nanotechnology TOHOKU UNIVERSITY World Premier International Research Center Initiative For AIMR Members Link Site map Copyright © 2020 Tohoku University. All Rights Reserved.
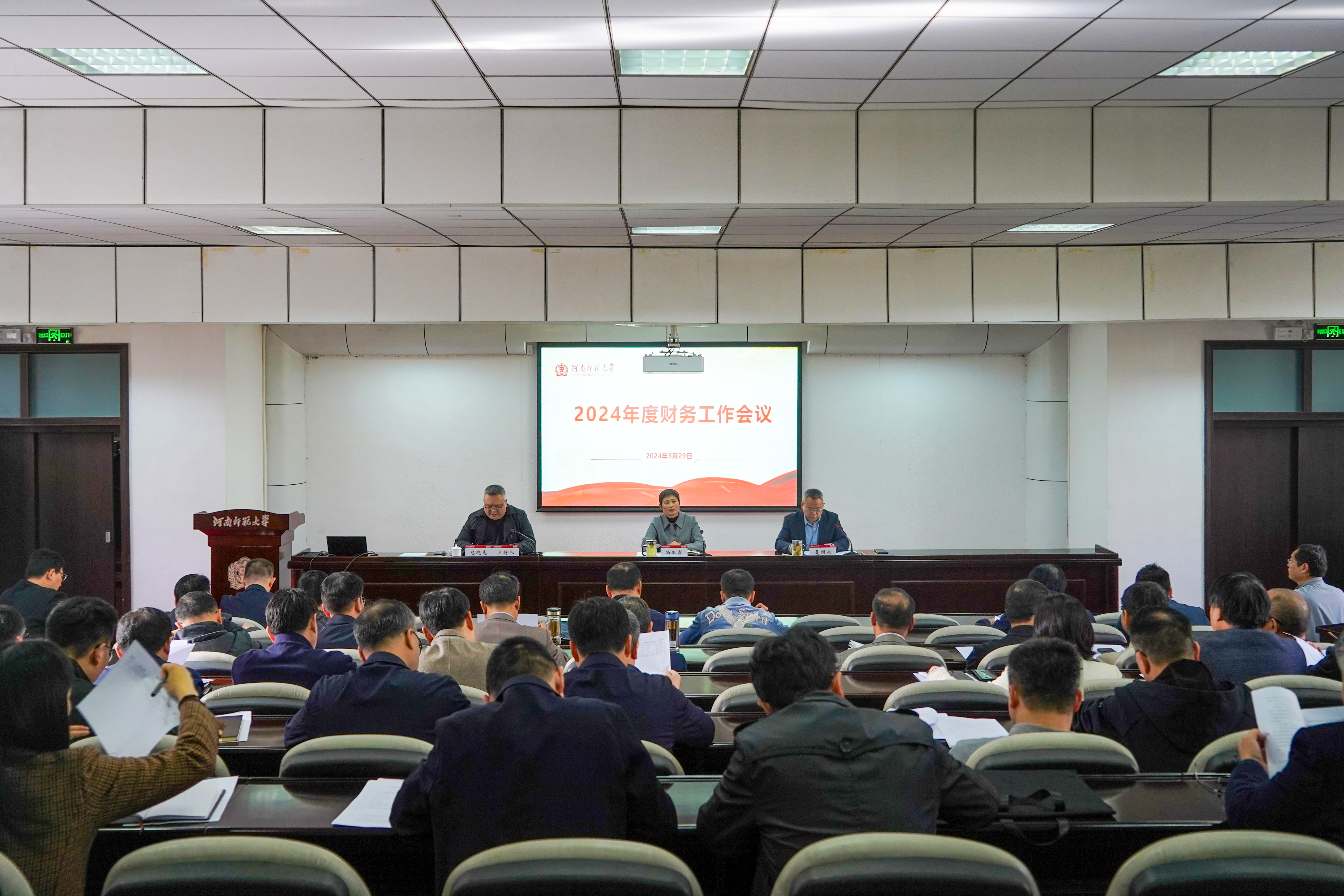